What is a recommendation in the era of artificial intelligence?
What happens when you choose a restaurant on the Internet? Or when you want to buy a book? Or find a song? Or get hooked on a new series? BBVA Data & Analytics in a new item for dissemination explains how the different recommendation systems used by Amazon, Netflix, and Spotify work and how they will extend beyond the consumer and entertainment sectors.
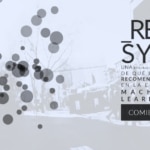
Internet users face so many options that web browsers no longer do the job. The human mind is not prepared to process the large volume of information now available, and Internet users look to the digital world to discover new possibilities. Using machine learning and its sub-discipline, deep learning, Artificial intelligence (AI), supports the creation of algorithms that replicate real-life logic used in decision-making when consulting friends or trusted experts about shared interests. The fundamental difference is that instead of asking one or two people, artificial intelligence makes it possible for us to get input from a group as large as an entire city.
“Recommendation engines are making it possible to take the leap from merely organizing information to offering personalized suggestions or advice according to specific tastes or needs in an automated way.” says Juan Murillo, BBVA's head of Analytics Dissemination. BBVA has already started down this path with services such as Baby Planner, Bconomy and Commerce360, a path that opens the potential to a new world of services aimed at helping us organize all aspects of our lives using real data as we weigh our decisions.
Data is the fuel
Recommendation engines, like all artificial intelligence systems, feed off data. The fact that AI is now more tangible than ever is precisely down to an increasingly complete digital fingerprint left by Internet users. Every interaction in social networks, on the web, or in messaging systems provides data that reveals tastes, trends, preferences, etc.
Big data enabled the processing of those huge quantities of data, whereas automated learning —or machine learning— “equips machines to learn and perform complex tasks without having been specifically programmed for them,” explains BBVA Data and Analytics’ Iskra Velitchkova, author of the Recsys study and specialist in Visual Thinking . “That's how motors that can make decisions based on a kind of concrete reasoning are created. This reasoning, when it’s automated, is what we call an algorithm.” points out Juan María Arevalo.
These algorithms are trained by the data to find patterns; they learn from them and are able to form new relationships. In the case of recommendation systems, they learn to find and choose new suggestions for each user.
Currently, most recommendations fundamentally work according to three models, which are explained in the Recsys study using a little test in which 109 participants were interviewed about their food preferences while placed in front of 30 international dishes.
Popularity
This model makes a recommendation according to the tastes of the majority. In the case of the example in the study, the most recommended food was paella, followed by pizza since these were the preferences chosen by a majority of the participants.
These systems don't take either the user or the product into account.
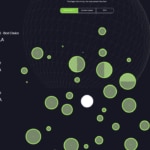
Content based
This type of algorithm makes recommendations based on user and product profiles (attributes, categories, etc). “Hence, these recommendations are made from key words, attributes, etc from the content.” affirms Marco Creatura, data scientist at BBVA Data & Analytics.
This kind of algorithm is frequently used when there is no history of page views, shopping, or use for the customer or the product to recommend. So, in Recsys’ example “for Chinese food, it chooses the most popular dishes from this cuisine: glazed duck, fried rice, spring rolls, etc.” Content-based methods are actually combined with algorithms that use collaborative filtering, which is explained below.
KNN (K-Nearest Neighbor)
The recommendations approach based on user history is known generally as collaborative filtering. From this history, similarities between users can be identified and used to generate a recommendation. This is what KNN does; it uses the (K) users most similar to a user in order to recommend a new product.
That's why, if you like guacamole, KNN recommends Spanish tortilla, pizza and pasta — those preferences of people who have similar tastes to yours.
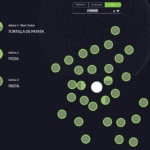
These are the most sophisticated recommendations, the ones that allow more personalized options. This is the system that is behind Amazon purchases or Netflix’s movie and series recommendations.
Less linear associations
In its Recsys article, BBVA Data & Analytics tells the story of a birthday party and summarizes the three ways these recommendation models are used to choose the restaurant: it’s a fashionable, popular place; it has the same kind of food that you usually eat; or people with a similar profile to you go there.
The challenge artificial intelligence now faces is being able to develop methodologies that can make less linear associations. Those that can bring thoughts together that have nothing to do with logic, but everything to do with experience and emotions.
Those thoughts that are triggered when you try a croquette that sparks memories of your grandmother and of summer vacations in the country and suddenly you have the bright idea of organizing a weekend trip to the countryside. All in a matter of seconds: These croquettes are delicious! How about going to the country for the weekend?
“The human mind makes non-linear associations, so two very different songs might recall one very vital moment in time, or a place might evoke something very concrete for someone who has never been there before. This is an enormous challenge for machines,” comments Velitchkova.
“We believe that recommendations have much further to go, to model the relationship between a person and the products they use. Today ‘deep learning’ is beginning to let us find spaces where people and products coexist, where they have a relationship, although it might not be obvious by using immediate logic.” adds Juan Arévalo, Data scientist at BBVA Data & Analytics and expert in recommendation systems.
In the Recsys article, BBVA Data & Analytics considers whether measurable data can be identified allowing algorithms to create these implicit relationships and make even more efficient and personal recommendations in all essential aspects of our lives, including of course, the financial.
New AI technologies are trying to move closer to this approach. Meanwhile, BBVA Data & Analytics has attempted to provide a graphical view that represents interactions organized in multidimensional fields that could end up giving order to a chain of thoughts such as ‘grandma’s madeleine moment’ triggered in Marcel Proust. An abstraction in the form of a story that unfolds from such a point of departure.
This project is part of BBVA's educational outreach, a part of its efforts to make the algorithms that impact people and society more transparent and clear.