BBVA Mexico and Clarity AI study consumption patterns in Mexico
BBVA Research through its Global Economic Research area and Clarity AI, presents the "Cash vs. card consumption patterns: a Machine Learning approach" study on the use of cards and cash in the country.
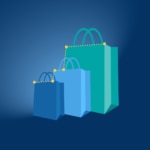
This is the first data analysis carried out in the country using machine techniques to identify the existence of non-linearities for determining usage patterns within different payment channels, in addition to the importance of financial development for consumer decision-making.
The study of household consumption patterns and payment channels is relevant for inclusive development policies, especially in countries such as Mexico, where 56 out of every 100 workers work in the informal sector. The identification of target variables on which public policies can have an impact to encourage the use of non-cash payment methods is of primary importance and ensures the rapid adoption of new technologies (such as CoDi) or a further deepening of traditional payment channels (cards).
The results of the study point to the income level of individuals as the main variable that determines the type of payment channel used most intensively. Lower income levels are associated with higher cash spending, and machine learning models find stronger non-linear relationships for the lowest income levels. This suggests that a positive shock to the income of the poorest households has a greater effect in terms of reducing the use of cash as a means of payment, compared to the effect of a positive shock to the income of households in the highest deciles.
On the other hand, in addition to income, the depth of the financial system and the urbanization level of the municipality determine more intensive card use; machine learning models identify stronger non-linear relationships for higher levels of financial inclusion (measured as the number of loans per 10,000 inhabitants). These results indicate that, in more urbanized areas, a positive shock in the number of people accessing financial services has the greatest effect on card use, compared to less urban areas with a smaller network of access points to the financial system.
The “Cash vs. Card Consumption Patterns: a Machine Learning Approach” study by BBVA Research and Clarity AI (Oliver et al., 2021) complements the analysis of the determinants of payment channel use with the identification of the type of goods and services that are most frequently paid for in cash and those that are most frequently purchased with cards. Most of the goods classified as "normal" were paid for in cash, including food, alcoholic beverages and tobacco. On the other hand, most of the goods or services classified as "superior" were paid for by credit card. These include travel, clothing, restaurants and education.
The findings suggest that the best public policies to promote the use of alternative payment methods should take into account the particularities of each region and/or municipality, and should not be approached as symmetrical and homogeneous policies throughout the whole country that do not take into account the particularities of each entity. In lower-income and less urbanized areas, cash usage will be reduced to a greater extent with the implementation of policies that favorably impact the income of the poorest households. In more urbanized areas with a larger network of banking access points, cash usage will be reduced with policies that increase the number of people using a banking instrument (credit/savings). The design of public policies should also focus on enabling alternative forms of payment as a priority in establishments that sell normal goods, especially food and beverages.
The study was conducted with the analysis of economists Álvaro Ortiz and Tomasa Rodrigo of BBVA Research; Saidé Salazar of BBVA Research Mexico; as well as Jaime Oliver and Ignacio Tamarit of Clarity AI.