When artificial intelligence helps you find out whether your app is helping your customers
BBVA conducted a study in Spain and Mexico that shows that customers who use its main financial health tools save 11 percent and 20 percent more, respectively. But how did the bank ensure that they eliminated the influence of other variables when calculating this savings? To do so, the bank used two machine learning models and causal inference, a statistical methodology that detects complex cause-effect relationships.
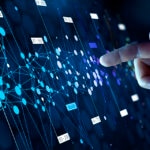
“Correlation does not imply causality.” This well-known statistics principle defines the different relationships that can be established between two facts. The correlation refers to the fact they are connected by some kind of association, while causality implies the extent to which one fact causes the other. A very famous example shows how easy it can be to confuse them. “When more ice cream is eaten, there are also more cases of drowning at beaches and pools. Therefore, eating ice cream increases the probability of people drowning.” This is an erroneous premise from a causal standpoint because the two facts are correlated, but there are other factors, such as the summer heat that increase the probability of them occurring.
Although it is very clear where the confusion lies in this example, in other cases it may be less evident. In its study, BBVA wanted to determine whether customers who use its financial health tools save more than those who do not. But there are certain correlations associated with this use that must be removed from the equation. Factors such as age (younger people tend to use apps more), income (the higher the level of income, the more likely to use all available options to manage it) and the level of spending (people who save more tend to use more savings tools) influence the choice to manage money through digital tools.
A method companies often use to assess the usefulness of their digital tools are A/B tests in which one group of users is given access to the features and another group is not. The results are then compared. But this methodology presented a problem for the study BBVA wanted to conduct, as its financial health tools are available to all customers on its app and website. The bank could not simply remove access to them for some people to test its hypothesis.
“Therefore, the Data team decided to use causal inference, the statistical methodology that makes it possible to work with complex causal relationships when controlled experimentation with other methods such as A/B tests cannot be used,” explained Roberto Maestre, Principal Manager of Data at BBVA Spain, and the leader of the teams that conducted the analysis. Causal inference requires the researcher to identify so-called ‘confounding’ variables that could influence the result and must be removed. In BBVA’s case, age, income, and spending level were established as confounding variables due to the reasons explained above. “Then, instead of analyzing individual profiles, we grouped two users with similar ages, income and spending levels in a set of ‘twins’. They were then subject to analysis because they were only different in that one of them used the app’s financial health tools and the other did not,” Maestre continued explaining. This pairing was completely anonymous as specific people were not grouped together, but socioeconomic profiles.
To carry out the study, the Data team analyzed each set of ‘twins’ with two different artificial intelligence models - a technique known as ‘double machine learning’. One of the models was dedicated to determining how the confounding variables influenced whether one of the members of each pair used the tools on BBVA’s app, and the other did not. With data from all sets of twins, the team obtained the comparative effect of how using the features or not depended on their age, income and spending level. In Spain, the analysis focused on three of the app’s features that are used the most: ‘Movimientos Previstos’ (Expected Transactions), ‘Mis Presupuestos’ (My Budgets) and ‘Objetivos de Gastos’ Spending Goals. Meanwhile, in Mexico, the following tools were studied: ‘Mi día a día’, (My Day-to-Day) ‘BBVA Plan’ and ‘Apartados’ (Sections).
The second machine learning model predicted how account balances (in other words, higher or lower savings) should vary among the different pairs based also on the confounding variables, and compared this prediction with actual savings.
The difference between the results of the two models eliminates the influence of age, income and spending level, and gives the savings rate that is due solely to the use of the tools. In Spain, it is 11 percent for the analyzed features and in Mexico it is up to 20 percent.
The results we obtain with causal inference are not the only measure with which we can see how a digital tool impacts users’ lives, as we also have to evaluate how much they use it and how satisfied they are with it,” Roberto Maestre said. “But without a doubt, when combined with artificial intelligence it is a very powerful methodology for companies to make our digital strategy much more effective.”