What is ‘explainable AI’? Taking the mystery out of technology
Machine learning has no end of business applications. Consumers and citizens stand to benefit from this technology, which lets them manage their financial health, streamlines their digital processes, or makes their online experience smoother across multiple platforms, among other uses. A basic premise has emerged from this boom: there is nothing mysterious or magical about artificial intelligence (AI), and nor should it seem so. For more complex algorithms, data scientists develop tools to help us understand how they make their decisions.
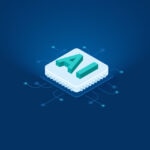
The concept of ‘explainable AI’ has been steadily emerging, so much so that a new Artificial Intelligence Act is currently on the drawing board at EU level. The Act states that this technology must be sufficiently explainable, among other characteristics, in order to build confidence in AI outputs. But what does that mean exactly?
An algorithm is considered explainable when it is possible to interpret and understand how it has obtained its predictions or results. This is a crucial feature, since these tools make their calculations based on vast quantities of interrelated data, and the calculations can be very simple or extremely complex.
The simplest ones can be understood by simply looking at how they work. A good example of this would be linear regression, which is based on simple mathematical and statistical equations to calculate the results; it is easy to understand how, and to what extent, the input variables influence the output variable. For instance, an AI can use linear regressions to, for instance, calculate the energy consumption of a house as a function of its size, number of rooms and geographic location.
Understanding an artificial ‘brain’
In contrast, AI models that perform more complex calculations are less transparent when it comes to understanding how they arrive at their results. This includes artificial neural networks and deep learning networks used in voice recognition methods, financial fraud detection, image and text generation, spam mail classification, risk assessment or sentiment analysis of text posted on social media platforms, among other applications. Because of this opaqueness, some of them are referred to as ‘black box’ models.
These models factor in a huge number of variables and the process followed to perform the calculation—similar to that of a human brain—is so complex that it cannot be made out. To get around this problem, data scientists have developed ‘explainability’ methods to pinpoint those variables that most influence decision-making in models, either at a general level or for specific cases; that is, why the results may be different for seemingly similar cases. Using these methods, scientists can determine whether the model is performing properly and therefore gauge its reliability.
One example of this toolkit is the explainability module contained in the Mercury code library, a compilation of algorithms that BBVA uses to develop its products and that the bank has released to the open source community. This module features various analytical components that developers can apply in order to understand the models used in various financial products, ranging from credit risk detection to the creation of recommender systems, among others.
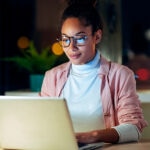
Explainability adapted to the user
The ‘explainability’ of the algorithm should be adapted to the knowledge of the different types of users who need it, whether the scientists themselves who work with AI, non-expert professionals or the general public. So say Andrea Falcón and Ángela Moreno, both data scientists at BBVA AI Factory who design machine learning algorithms applied to the bank’s products. These include tools capable of spotting significant events or activities among customers when using the BBVA app, or recommender systems that suggest courses of action or possible products that could help users improve their financial health.
“When explaining a prediction to another data scientist, we need to tell them how we go about measuring the way the model delivers its predictions,” says Andrea Falcón. “Conversely, when we need to explain it to a colleague at a given department—who certainly knows their product and the customer they are targeting, but has no real technical knowledge—our explanation will focus on the specific characteristics of the products or customers that make the model propose one thing or the other.”
Her colleague Ángela Moreno gives a practical example: “Let’s imagine two clients who have the same age and income, yet the model recommends different actions for them in response to the same event. For example, when they receive a windfall, the model advises one of them to send it to their savings account but suggests to the other that they use it to pay off a loan. “Here, it’s plain to see that other variables are in play, beyond their age and income, so explainability techniques can help us understand what variables the model is looking at when suggesting the different actions.”
Building trust in AI
“What do we lose out on if we fail to explain?” muses Moreno. “First of all, the model development team would be unable to detect certain errors or malfunctions, or confirm assumptions about the model. Also, it’s a way of bringing advanced analytics closer to our business colleagues, who are not data scientists and need to trust that a machine learning model is more useful for clients than other more rudimentary systems that were used in the past.”
Falcón also exclaims that “by reviewing the calculations and, more precisely, how the different variables interact and influence the predictions, we can identify possible discriminations that need to be corrected. Ultimately, this helps us understand why the model makes a given decision for one client, but a different decision for a seemingly similar client.”
Therefore, understanding how different AIs use data to make their decisions helps data scientists improve algorithms if they detect that they are failing to use data correctly, and product managers at companies to continue tailoring them to the needs of their clients.
Meanwhile, sharing this knowledge with the general public will help users understand how AI uses their data and reassure them that this process is always supervised by a human to avoid any deviation. All this helps to build trust in the value of technology in fulfilling its goal of improving people’s lives.