What are PET technologies?: how to maximize data value while preserving privacy
Is it possible to take advantage of big data without it affecting the privacy of users? The answer may reside in “Privacy-enhancing technologies”, or PETs, a set of technologies that use different computational and mathematical approaches with the same purpose: extracting data value in order to unleash its full commercial, scientific and social potential, without jeopardizing the privacy and security of this information.
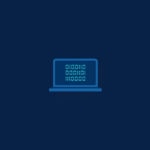
For a virtual assistant, like Siri or Alexa, to recognize their owner’s voice, each data that could possibly be collected will be of great use. The more data they analyze, the better they will become at foreseeing the user’s needs and providing personalized answers, thanks to “machine learning”. Although this may come at a price: the privacy of the individuals who feed the system with their data to make it increasingly intelligent.
You don't have to go far to find clear examples of this problem. From autonomous vehicles, to the field of healthcare or the energy industry, the same situation could be applied to innumerable scenarios in which advanced data analysis has great advantages (for users, institutions and society as a whole), but at the same time, it opens up new scenarios in which privacy, anonymity and data security are put at risk.
“This situation is leading to growing concern among the general public and increasingly strict regulatory pressure, which limits what companies and institutions can do with big data,” explains Iván Moreno, R&D Manager at BBVA New Digital Businesses (NDB). Given this situation, the NDB Unit has been researching a series of cryptographic methods that allow data to be analyzed and shared without exposing their content to third parties. They are called PETs, meaning “Privacy-Enhancing Technologies”, or techniques for improving privacy, described under this definition in a recent report of the World Economic Forum where its role in the financial sector was analyzed.
How do they work?
Specifically, the technologies encompassed under this umbrella term, and in which the NDB Unit is researching applications for the financial sector, are:
- Homomorphic Encryption
This technique allows operations to be performed on encrypted data so that the results are the same as if they had been performed with data that is not encrypted. This way, a company can share data with another for analysis purposes, without ceasing to be totally anonymous and private, "since they would only have it in an unintelligible format," Moreno explained.
Its practical applications are limited to the volume of data, since it can only be used to operate with limited amounts of information.
- Secure Multi-Party Computation
This cryptographic technology is actually a subcategory of the previous one, which allows complex computational or analytical operations to be performed on a larger volume of encrypted data; which in turn allows models of “machine learning” to be applied to them.
Its use is already widespread in companies such as Google and Facebook, and is present in products such as the machine learning Tensor Flow tool, which enables models to be trained with encrypted third-party data. For this purpose, companies share their encrypted data with a third party, who analyzes it and sends back the results of the analysis, without compromising the privacy of its content.
One of the fields with the most evident application for this is healthcare. “There are already projects that improve diagnosis by using image analysis based on this technology, so that the systems can learn while keeping the patients’ private data from being disclosed”, added Moreno.
- Federated Learning
This method goes one step further than the rest, and it enables automated learning models to be trained from data that does not even have to leave the company or the device it was generated on. A very useful approach in the fields of the Internet of things and in the advanced analytics one.
This technology, which large companies such as Google are already researching, could also help, for example, to train the intelligence systems of virtual assistants by collecting data on site on the different devices connected to a collaborative learning network, but in a way that keeps this data from leaving the device on which it is generated. “The only thing that is shared is the data generated that will be relevant for the model’s training, without any personal or sensitive data, which helps the learning system grow but does not contain users' private information,” stated the NDB researcher.
- Zero-Knowledge Proofs
This technology allows to verify whether there is validity to information, without exposing the data that proves it. This is possible thanks to a series of cryptographic algorithms through which a “tester” can mathematically prove to a “verifier” that a computational statement is correct, without revealing any data.
Its applications are numerous for the creation of opportunities in the banking and insurance sectors, in which it could facilitate access to products or services for which private customer information is required, while ensuring that they do not expose their data.
- Differential Privacy
This cryptographic system allows a “random noise” layer to be added to a data set, so that it is impossible to extract specific information about each individual piece of information. Thus, it is possible to share the results of applying an automated learning model to a data set with a third party, while keeping the analyzed data private.
“At NDB we have assessed the possibility to use this technology together with federated learning, so that various companies or organizations can benefit from collaborative learning models without jeopardizing the privacy of the data that they are working with,” Moreno explained.
New Opportunities and Unexplored Pathways
The NDB Unit has various research approaches to explore and perform tests with these technologies, particularly with federated learning, due to its potential to create new business models that can be included in “a new economy based on data”, combined with other technologies, such as artificial intelligence and the Internet of things. “Which, currently, face many obstacles when it comes to developing their true potential because of the regulation´s restrictions, especially in privacy” the researcher added.
“We can find out things that we had not even imagined were possible thanks to new data combination variables ”
One of the most interesting lines of research analyzed, through various concept tests, was the creation of collaborative learning models in which several companies or organizations, even from different industries, can contribute with their respective data in order to apply machine learning models to it, without violating its privacy. “This way we can find out things that we had not even imagined were possible, thanks to new data combination variables from different fields and industries,” he added.
Thanks to the new collaborative models, mechanisms could be created for companies or organizations to obtain benefits in exchange for the extraction of data value from different data sets. This could include data of all kinds, and not necessarily of a personal type; for example, that derived from the use of bank cards, to improve fraud detection or online services models, in order to improve cybersecurity. “The more entities contribute with their data to understand all the types of fraud that exist, the better models can be created collectively to detect it,” Moreno explained.
In turn, this new type of approach poses some challenges, which have already been analyzed by NDB. “We would need to create systems to determine how much each of the organizations is contributing to the learning models, based on the type of data each of them provide, however, once again, without disclosing the content of the data itself,” he noted.