What are neural networks? Applications, types and examples
Neural networks are transforming technology. Natural-language processing, voice recognition and artificial vision are just some of the applications of a sector that will be worth $1bn by 2030. The technology, first conceived decades ago to emulate the human brain, has boomed in recent years.
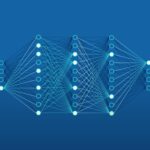
Neural networks power Google's search engine and ChatGPT. This technology has boomed recently and will continue gaining popularity, with the neural network market expected to grow by 21.4% from 2023 to 2030, reaching $1.02 billion (€939 million), according to SNS Insider's ‘Artificial Neural Network Market.’ Since their origin in the 1940s, neural networks, which enable computers to process data like the human brain, have found numerous applications.
What are neural networks?
Neural networks were first proposed by Warren McCullough and Walter Pitts in 1944. However, it was not until the 1980s that English psychologist Geoffrey Hinton significantly advanced them through his research in deep learning, a branch of artificial intelligence that enables machines to learn from nodes that emulate neurons. In this framework, the network functions as the brain.
Deep neural networks are trained using large datasets from which they extract patterns to make predictions. Multiple layers of nodes work together to analyze the data and produce a predictive result. Unlike machine learning, where data is preprocessed, deep learning eliminates this step, allowing neural networks to independently learn and identify patterns from the initial information.
Applications of neural networks
The development of neural networks has allowed computers to make decisions without human intervention, leading to a wide range of applications. As early as the 1980s, Hinton was working on applications such as speech recognition and machine vision. With recent computational advances and the explosion of data, neural networks have experienced a boom and now have numerous applications.
- Natural language processing. Natural language processing (NLP) enables computers to interpret and respond to written text. Its uses include engaging with chatbots and virtual assistants in banking, as well as analyzing, classifying, and summarizing information. Additionally, NLP allows for the creation of original texts through large language models (LLMs) trained on extensive datasets. GPT-4, the LLM that powers ChatGPT, exemplifies this technology.
- Voice recognition. Machines can recognize spoken text, detect the language, and analyze the tone used. This capability facilitates transcribing conversations, as Pinpoint does, generating automatic subtitles, as Veed.io does, and classifying customer telephone requests by service needed.
- Machine vision. Machine vision enables computers to extract information from audiovisual documents. This is particularly useful for developing self-driving vehicles, where it is essential to recognize signals, understand their meanings, and detect surrounding obstacles. More common applications of machine vision include facial recognition, as with Apple's Face ID, and detecting potentially offensive audiovisual content. The BBVA Foundation also supports research in this field to enhance security through video analysis using neural networks.
- Generating recommendations. Recommendation systems track a person's online activity to provide personalized suggestions. This is particularly evident on social media, where relevant products and services are offered to users. Instagram and Netflix employ neural networks to present users with the most pertinent content. Another example is BBVA's expense forecasting app, which enables users to monitor finances in real time and automate savings through one or more rules.
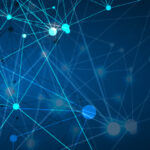
Types of neural networks
Since their invention, different types of neural networks have been designed. The most common by use are convolutional neural networks and recurrent neural networks, as IBM points out.
- Recurrent neural networks (RNNs), invented in the 1980s, can process sequences of words. Their development hinged on technology capable of retaining sequences of previously analyzed words for further learning. RNNs run on feedback loops, which send information backwards to verify the accuracy of predictions and learn from errors.
- Convolutional neural networks (CNNs) consist of multiple interconnected layers that deconstruct, analyze, and categorize images and videos. Each layer focuses on specific aspects of the image, meaning that more layers allow for more complex analysis. CNNs are crucial for advancing machine vision and are trained with vast numbers of images.
Machines now have the ability to engage in casual conversations with us and recognize our face and voice. Neural networks, a technology developed decades ago to mimic the human brain, are becoming ubiquitous in the digital realm.