What AI algorithms does BBVA use to boost its customers' finances?
Neural networks, natural language processing, machine learning... AI is an essential partner for BBVA to help its customers manage their savings, manage their investments, analyze their consumption and plan for their next outgoings.
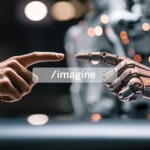
BBVA customers are increasingly using the bank's app and website to track spending, make informed financial decisions, and boost savings.
In Spain, six out of ten digital users leverage these features, with satisfaction rates nearly 24% higher than non-digital users. Spanish customers interacted with AI features 70 million times in Q1 2024, a 23 percent year-over-year increase. The global data is even more striking: during the first quarter, there were 391 million interactions with these features across all the countries where BBVA is present, 49% more than in the same period in 2023.
This strong adoption highlights AI's potential in personal finance. BBVA's data scientists use advanced algorithms and AI models to enhance these financial health features, driving higher engagement and satisfaction.
Classification of financial transactions
BBVA's app and transactional website categorize banking transactions into spending, income, and savings/investment, including accounts from BBVA and other banks upon request. Customers can manually adjust these automatic classifications, applying changes to future transactions.
This allows BBVA to offer personalized advice, such as "reduce your spending in bars and restaurants by 10 percent," instead of generic suggestions. Since June 2023, in Spain, credit card transactions are classified in real time, showing customers how each expense impacts their income and its category immediately. Manual reclassifications by customers also apply instantly.
BBVA's financial transaction analysis
BBVA account and card transactions are classified to one category or another based on certain attributes. The name of the business, its business activity code, the details of the receipt, type of transaction, etc., allow identification of whether it is a payroll entry or an expense for food, fuel, transportation or clothing, for example.
"To classify these transactions that have such a fine level of detail, it is sufficient to use algorithms in which we know in advance what the result will be, known as deterministic algorithms," says Maria Ruiz, an Advanced Analytics data scientist at BBVA's Client Solutions. " These are most suitable when we have data with a high level of detail, we want to prioritize the explainability of the algorithm and ensure that the classification makes sense," Ruiz adds.
Analysis of other banks’ transactions
BBVA’s aggregated transaction data from other banks is less detailed, typically including only the bank identifier, transaction type, amount, and a variable description. "With such inconsistent data, we needed a model to infer categories from descriptions," says María Ruiz. BBVA developed a neural network model, inspired by brain processes and adept at natural language processing (NLP) tasks.
Data scientists train the neural network using samples to learn term-category relationships, such as 'Zara' for 'clothing store' and 'college' for 'schools.' The model also handles abbreviations ("transf" for "transfer") and grammatical variations ("pay roll" for "payroll").
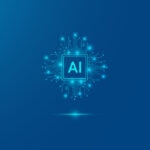
Adapting financial health proposals
To increase the effectiveness of communication, it is important that proposals and suggestions for improving the customer's financial health are tailored to the customer's situation and interests.
Customer segmentation
BBVA uses advanced analytics to identify groups of customers with similar needs in order to tailor the financial health plan to each individual case. "This segmentation, developed with experts, helps to target the most appropriate proposals to each person based on the estimates we have about their financial health," says David Muelas, data scientist at BBVA AI Factory.
In this way, the bank creates groups of customers defined on the basis of certain key factors (such as financial cushion or savings capacity) to reinforce the financial health actions and experiences that best suit their objectives and situation. For example, by making tools such as Budgets more visible to customers who are struggling to make ends meet.
Understanding customer feedback to improve financial health suggestions
BBVA customers can leave detailed comments within the financial health features, analyzed in the aggregate by the bank’s data scientists using NLP techniques to identify improvement areas and refine personalized proposals.
Previously, manual comment analysis was labor-intensive, needing differentiation between simple messages like "All good" and detailed feedback like "I like being notified of higher-than-usual payments." Advances in language models now streamline the extraction of valuable insights, efficiently identifying positive and negative perceptions, says Muelas.
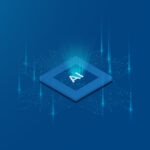
Predicting financial health changes over time
BBVA's app and website help users anticipate and estimate future income and expenses, improving personal finance management. Customers can view expected transactions for the current and next month.
Using ensemble machine learning, which combines multiple models, BBVA predicts future transactions, their amounts, and dates. Data scientists have created a catalog of fixed expenses like subscriptions and analyze transaction patterns statistically to detect other regular costs, such as gym memberships.
Forecasts also identify financial events that could impact customers, such as higher-than-expected bills. The mobile app checks predictions against actual transactions, alerting users to unexpected events, amount deviations, or missed expected transactions.
Predicting account balances
BBVA has also developed an account balance prediction model that allows estimating its future evolution and detecting out-of-the-ordinary values.
This model uses a neural network to provide predictions at different probability levels. In addition, it has been trained in such a way that it is able to generate long-term predictions that reflect the expected evolution and variability of the balance. "Thus, when we observe balance values that are far from what is expected taking into account the uncertainty of the prediction, we can warn clients and suggest possible actions to respond to the events that have caused them, if necessary," emphasizes David Muelas.