Concepts such as big data, machine learning, artificial intelligence and data science are increasingly at the center of the debate about the digital revolution. But why is everyone talking about them? Do we really understand these concepts, or the implications they have on our evolution as a society?
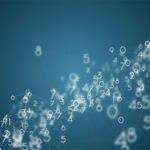
Elena Alfaro, Head of Data Strategy & Data Science Innovation at BBVA and a founding member of Spain´s Group of Experts on Artificial Intelligence and Big Data, uses real examples to describe the opportunities these new capacities present, and the responsibility and the dilemmas they imply. She addressed these topics in an article in the Economy Ministry’s magazine ICE, which we recommend reading in full.
We’d like to start the New Year with a strategic reflection that summarizes the ideas Elena put forth in that article, and in speeches she gave during the past year.
Why now?
There are three main reasons, or trends, behind the revolution that has taken place in the past five years:
- The digitization of information. Everything we do today leaves a digital footprint. Whenever we access an application, make a payment with a bank card, read the news online, pass by a security camera or buy a ticket for public transportation, we create data that indicate what we've done, where and when. This means that in the digital world, there is an increasingly exhaustive record of what occurs in the physical world.
- Moore’s Law and its implications: the democratization of access to infrastructures that are increasingly affordable and which put the computer power required to instantly handle millions of interactions, within everyone´s reach. Processing and storage capacity have ceased to be a privilege of big corporations alone. There are no longer barriers of access to the hardware required to allow ideas to prosper in the digital world. Access to data—by virtue of having information about millions of interactions—is the only competitive advantage remaining. However, that access is worthless if it cannot be transformed into a real use for whoever generates the data.
- The increasing demands of customers and users, who are unwilling to wait for what they want; they seek products and services that are available instantly and customized to meet their needs. Data gives a voice to customers’ needs, and companies who are unaware of them will be flying blind and as a result, run into obstacles
Just as nothing can escape having a digital record, no business can elude the wave of digitalization and disruption.
Who is best positioned in this situation?
The three aforementioned trends create a scenario in which only data-driven companies will survive. Companies that make data the center of their strategy, and use data to learn from every interaction with the user, will be able to offer surprising, customized products and services. Some examples of companies that use data to create automatic, decision-making engines are: Amazon, with its collaborative filter algorithm, “people that purchased X also bought Y”; Netflix, thanks to its film and series recommendation system. LinkedIn, whose “People you might know” function, made the number of people who connected to others grow exponentially; Spotify, with its Release Radar, “Discover Weekly”, and “Daily Mix,” customized services for discovering new music as a function of the user’s tastes; and the travel search engine Kayak, whose price forecaster uses a time-series analysis to recommend whether to buy a plane ticket now or wait for the price to decline.
So what exactly does “data-driven” mean? On the one hand, it means making data-based decisions, ones that are not the result of intuition or hierarchy. It also means being capable of creating automated and customized digital products, through algorithms that improve the results obtained by direct human intervention. Those products can then be offered to millions of clients, often in real-time, in order to respond to customers’ new expectations and give them unique and immediate experiences.
From Big Data to Artificial Intelligence
The examples we’ve cited are leveraged by the tools that Data Science puts within our reach to resolve the problems of classification, comparison, prediction, optimization, recommendation and rating. When we have a large amount of data about the phenomenon we are studying, ideally a machine will process the results, managing vectors of many more dimensions than the human mind can conceive. This is called machine learning. What does this have to do with artificial intelligence?
Unfortunately, the term “artificial intelligence” does not describe something very specific; it has maintained the same mysterious aura since it was coined in the 1950’s. It refers more to future aspirations, by implying a certain “consciousness” in machines, which is a misinterpretation of the term. Why does this happen? Because once artificial intelligence is applied, it changes names.
For example, processing natural language, logistics optimization, recommendation engines, dynamic pricing, etc., are all examples of artificial intelligence. Therefore, the most precise way to define artificial intelligence today would be “that which helps us to resolve a problem automatically and on a massive scale.” We are already seeing many artificial intelligence systems in operation; their appearance is far removed from the stereotype of the anthropomorphic, conscious robot, an image far removed from reality.
* This is the first article in a 3-part series exploring the challenges and opportunities of Data in the digital world. You can read the second one and the third one here.