The future of AI: can machines think?
Artificial intelligence systems that are capable of reasoning, creating art or even learning to teach other machines. These are a few examples of what could occur in the future with advances in deep learning. Three experts in the AI field shared their vision, concerns and hopes regarding the development of this technology during the launch of BBVA AI Factory.
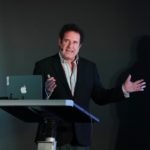
On February 24, 1956 a computer program designed by Arthur Samuel, IBM researcher, beat a person at checkers. The event was televised and the audience watched one of the first battles between man and machine with a mix of fascination and fear. It has since become a cultural icon.
It wasn’t long before this episode of history would repeat itself in other formats: from chess (Russian chess grandmaster Garry Kasparov lost at chess in 1997); backgammon and the Chinese game Go, to some machines learning on their own to play better than any human. And why this obsession among artificial intelligence researchers with board games? “Machines are very good at solving these problems because the computer doesn’t need to understand the rules of the game to win - it just has to see lots of examples of how to win a game. It needs data,” says Hod Lipson, Engineering and Data Science Professor at Columbia University in New York during the presentation of AI Factory, where he shared his vision of the past, present and future of artificial intelligence with BBVA’s new team.
As Lipson explained, it was in the 1950s and 1960s when the foundations were laid for machine learning - a discipline within artificial intelligence that imitates some aspects of human biology in order to teach machines to solve certain problems through experience. The research on artificial neural networks, however, was at a standstill for nearly 50 years due to the absence of two elements that were essential to advance further: computing power and the availability of large amounts of data to train the models with sufficient example
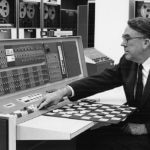
On February 24, 1956, Arthur Samuel’s Checkers program, which was developed for play on the IBM 701, was demonstrated to the public on television. (Source: IBM). - IBM
The return of machine learning
“Starting in the 1990s, however, this changed. With the new abundance of data generated by digital society, advances in machine learning have skyrocketed in recent years,” notes Lipson. Camaras, sensors and all types of devices are constantly capturing millions of structured data that serve to train these types of systems, which has exponentially refined their learning capacity.
Thanks to the vast amount of data available from the Internet, in just a few years of research, image recognition software surpassed humans in distinguishing one face from another, for example. This was possible thanks to the emergence of deep learning - a subcategory of machine learning that allows machines to not only learn from examples, but use data to actually train themselves to do so better and better. This technology is what is also driving advances in autonomous vehicles in recent years, “which are finally able to distinguish a puddle from a pothole” thanks to this refinement.
"Machine learning systems already exist that can design much better antenas than any antena ever created by humans”
Proof of this exponential growth is the growing demand for the discipline in universities and academies, as the Director of IBM Research, Darío Gil, explained during the event. “15 years ago no one studied it and now they barely fit in the classrooms,” he noted. Despite these advances, during his presentation, Gil discussed what he considered the next frontiers for artificial intelligence. On the one hand, the concept of “broad artificial intelligence”, which is capable of solving all types of tasks, versus “narrow artificial intelligence” like the artificial intelligence that currently exists, and is capable of solving “very specific” tasks “very well”.
We do not yet know when broad artificial intelligence will arrive. This will be difficult to accomplish with deep learning techniques alone. According to Gil, this discipline is not efficient at teaching machines to solve complex problems that involve the need to “reason and manage ambiguity” like humans do. “To do so, logic and reasoning must be combined, and machines must be capable of going beyond the rules,” he explained.
To reach this point, Gil maintains, one possible path forward entails the combination of machine learning techniques with symbolic artificial intelligence, a trend that stopped advancing in the 1980s and was based on giving programs a “representation system symbolic of thought”. Thus, according to Gil, the next steps in artificial intelligence will involve the creation of systems that are capable of really reading and understanding a text - “not processing it” - systems capable of writing their own artificial intelligence programs” and “systems capable of experimenting on their own,” he explained.
Creative machines with thoughts and feelings
Similarly, Hod Lipson described what he sees as the next challenges for this technology to overcome. One of them is to develop “creative machines”. “Machine learning systems already exist that can design much better antenas than any antena ever created by humans,” he said. There are also machines that are capable of designing proteins using deep learning algorithms with tremendous potential to advance research on diseases and develop vaccines.
According to Lipson, these technologies are also giving rise to a new form of art. Art that is born from a new type of perception - that of machines. “What will happen when machines see colors we don’t and can represent them?” he asked. The artistic aspect is interesting, he noted, but less important than what is actually behind this nearly unlimited ability to create: “Currently, a great deal has been invested in creating programs that develop the next generation of artificial intelligence,” he stressed.
Finally, Lipson raised the challenge of creating machines with “feelings” - machines that not only perceive their environment, but which are aware of their own body. To explain the advances taking place in this field in his laboratory, he showed the example of a “blind” robot that thanks to deep learning, was designed to learn to perceive the shape and capacities of its own body just using data obtained from sensors on its exterior. In a video, he showed the robot’s efforts to understand that it had four legs, and its ability to learn to walk based on this information. After losing one of its legs, the robot also demonstrated surprising resilience, reformulating its learning and managing to walk again with just three legs.
AI that explains itself
Understanding what occurs inside the “minds” of these machines with an increasing ability to learn is another one of the most complex challenges facing this technology. “The biggest problem of machine learning is the systems’ opacity,” the CTO of the Californian company NTENT and Director of Northeastern University’s Data Science Program in Silicon Valley, Ricardo Baeza-Yates, explained at the event. During his presentation, he explained what “explainable artificial intelligence” is: a trend that seeks to make the “black boxes” created by machine learning more transparent in order to prevent the perpetuation of possible biases present in the data used.
To illustrate the problem, Baeza-Yates used the example of image recognition software. “When it processes the image of a cat and decides that it’s a cat. What does it base this on?” he asked. Normally, an algorithm of this kind only offers a result (“this is a cat”), but not an explanation. To address this, according to the expert, the system must be able to offer a justification that helps researchers understand what process it used to make a decision. For example, it is a cat because it has ears, hair and a particular shape that corresponds to that of a cat.
However, the explanations are not always that simple. What happens if the cat is in a position where the system cannot identify any of these recognizable features? “Normally, the algorithms are afraid to say, ‘I don’t know,’” noted the researcher, so it would probably try to find another result instead of offering an ambiguous response. Nevertheless, Baeza-Yates says that this simple possibility could take researchers one step further in better understanding where the limits of these algorithms lie, and making artificial intelligence more transparent and human.
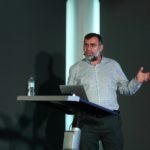
Ricardo Baeza-Yates, during his intervention at the event in BBVA AI Factory's new headquarters.