Responsible AI: Why do we need guardrails for artificial intelligence?
Guardrails are mechanisms to guide the responsible development of AI so that it does not become a technological, social, or security threat. Classical and generative AI require different types of guardrails, as the latter’s ability to engage directly with end users has introduced a whole new set of challenges for developers—such as the mass production of fake news or the creation of new methods for perpetrating cybercrime.
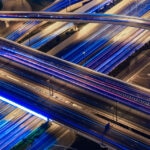
Just as road guardrails stop a vehicle from veering off the road in the event of an accident or driver error, AI development includes its own mechanisms to ensure that the technology remains within ethical, legal, and safe boundaries. These mechanisms also help counter the performance degradation that AI models can experience over time, often caused by shifts in input data or a lack of regular updates.
Their role is especially important amid the current business landscape, where the use of AI is now widespread. According to McKinsey’s latest The State of AI report, since 2021 there has been a 22 percent increase in companies using AI in at least one business function, while the number of organizations using AI across multiple functions has doubled, rising from 31 percent to 63 percent.
AI can pose varying levels of risk. The European AI Act, approved in 2024, classifies these risks as follows:
- Minimal risk: These systems are not subject to specific obligations under the Regulation. Examples here include AI used in video games or email tools used to filter out spam.
- Limited risk: These systems must meet transparency requirements. For instance, if a customer service channel is run by a chatbot instead of a person, the company must clearly inform users.
- High risk: This includes AI systems that could affect people’s health (e.g. surgical robots), safety (e.g. transport systems), or fundamental rights (e.g. software that screens CVs during recruitment processes). These systems are subject to strict requirements when it comes to quality, transparency, and human oversight.
- Unacceptable risk: These systems are banned outright because they pose a threat to people’s safety, livelihoods, or individual rights. The AI Act prohibits technologies enabling manipulation or deception, emotion recognition in workplaces or schools, real-time biometric identification in public spaces, or data scraping from the internet or security cameras to build facial recognition databases.
The development of high-risk AI systems under the new regulation must include both technical and organizational safeguards to protect fundamental rights. This means making sure that AI systems do not pose a threat to people’s safety, respect data privacy, avoid bias and discrimination, minimize errors and hallucinations (responses not based on real facts), and are secure against technical vulnerabilities. Furthermore, for both high-risk and limited-risk systems, the AI Act requires transparency—for example, clearly informing users when they are interacting with an AI system, or when technologies such as emotion recognition or synthetic content generation are being used.
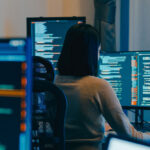
Some guardrails that could complement the European regulation include:
- Technological guardrails: These include automated bias detection systems, content moderation tools to flag hate speech or fake news, and security filters to prevent adversarial attacks, or to block illegal, violent, or sexual content on platforms accessible to minors. This category also includes constant monitoring tools to observe AI system behavior and perform robustness tests, and technical auditing systems to ensure explainability—that is, understanding how the AI reaches its conclusions.
- Procedural guardrails: The development of AI systems should comply with a company’s internal rules and ethical guidelines. Organizations must also establish clear policies and processes to review and approve AI systems before they are deployed, thus enabling them to identify flaws and vulnerabilities before they reach users or employees.
- Human guardrails: These involve direct human oversight, sometimes referred to as ‘human in the loop’—especially when systems impact people’s lives—and expert or ethics committee reviews to assess risks and make decisions about specific AI applications.
At BBVA, the detection of vulnerabilities and blocking of cyberattacks targeting the ‘Blue’ chatbot includes the use of technological guardrails, real-time monitoring, external cyberattack simulations (known as ‘AI red teaming’), and adversarial testing to evaluate system robustness and detect possible manipulations. “All these techniques help prevent fraud, data leaks, biased decisions, and regulatory issues, thus ensuring the integrity, confidentiality, and security of our clients’ financial data,” explains Juan Arévalo, Senior Manager at BBVA’s GenAI Lab.
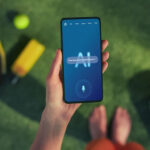
New Guardrails for Generative AI
Setting guardrails for classical (analytical) AI tools, such as non-generative machine learning, is relatively straightforward. These systems typically produce scores or probabilities, so many of the safeguards involve pre-established thresholds. For example, if a weather model predicts pressure, the output must always be a number greater than zero. However, the virtually limitless capabilities of today’s generative AI algorithms make it essential to guard against unpredictable or discriminatory outputs. It’s also crucial to limit their misuse, such as generating fake news at scale or enabling cyber fraud techniques like phishing, smishing, or CEO fraud via social engineering.
This is why developers must implement specific guardrails for generative models. Examples include watermarks and metadata embedded in AI-generated images, indicating that they have been created or edited by AI; and tools to ensure generative models do not execute self-written code without permission, or that their responses are not used to automate critical processes.
Another key aspect is ensuring the quality of the data used to train generative models. It is essential to have mechanisms that confirm the data is appropriate, relevant, and secure—and that the model is trained solely for its intended purpose, to avoid unintended responses or applications. For instance, the Blue chatbot is trained exclusively to respond to questions posed by BBVA customers related to banking transactions or their financial position. “In generative models, data quality is as important—if not more so—than in predictive ones, because it affects not only the accuracy and reliability of outputs, but also whether the model stays within its intended use,” explains Víctor Peláez, Discipline Leader for Governance and Regulation on BBVA’s Analytics Transformation team.
As models become more advanced and powerful, they tend to generate fewer hallucinations and become less vulnerable to attack, says Juan Arévalo, although human supervision will always remain essential: “In cybersecurity, for example, we’ll never be able to predict every possible attack,” he explains. “It’s up to humans to detect new threats and vulnerabilities, adapt the guardrails accordingly, and make sure this process is part of the AI system’s development lifecycle.”