How to make artificial intelligence more ethical and transparent
Along with the promise it bears, artificial intelligence also brings with it a number of risks that need to be kept in mind and addressed. What measures are data scientists taking to avoid information fed to machines from being incomplete or biased?
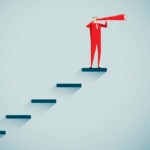
Artificial intelligence (AI) is often defined as a field of computing that creates systems able to perform tasks normally requiring human intelligence such as translating documents, driving a car and recognizing faces. But what happens when machines, like human beings, make mistakes? Can an AI system be biased? Do they mirror the same prejudices as humans when making decisions?
Such questions acquire increasing importance in a context in which AI is beginning to form part of the day-to-day existence of companies and individuals. All the more so when a number of eye-catching cases have entered the arena of public debate underscoring social concerns about the transparency of algorithms. From bots that have learned to speak in an inappropriate, even racist, manner of other Twitter users, to regrettable errors in interpreting data of images. These are examples of how systems based on machine learning can have disastrous consequences when the data fed to the machines is mistaken
“Models based on data can reflect the inherent biases of the sources used to train them and can mirror and even compound them”, explains Roberto Maestre, senior data scientist at BBVA Data & Analytics. “How then to ensure this does not happen? For the BBVA expert there are basically two ways: algorithmic transparency and the incorporation of measures to control such biases in the models themselves.
Algorithmic transparency
One of the problems facing these scientists when assessing the transparency of AI is the known dilemma of the ‘black box’ which often makes it virtually impossible to know the path taken by an AI model to arrive at a certain conclusion or decision. Maestre explains that this should not be used as a defense to eschew responsibility for one’s actions. Work is required to identify, as far as is possible, the variables that weigh most in the decision that is taken and to make this information available to the public.
"It is our duty to be aware, control and mitigate the biases existing in the training data"
In order to make machine-learning systems easier to grasp and more accessible, Maestre also emphasizes the need to “establish a common language” that can be used by the scientific community. He recommends peer review of work carried out through publication in an environment conducive to discussion and correcting models. “This discussion should be seen as a scientific review open to people with different profiles, not just AI experts, to gauge the impact on decision-making when designing algorithms”, he adds.
This process, which has been described as “algorithmic auditing”, implies at the same time undertaking a systematic review of work that allows all processes to be documented and to establish review mechanisms that assign a “quality guarantee seal” to models.
Control measures
At the same time, it is necessary to integrate specific measures to control possible biases originated by the AI itself. Firstly, you need to “define and identify” the biases that can arise with the data to be used to feed the algorithms. “Once this has been done, you need to propose specific measures to quantify the impact”, Maestre adds. Once identified, the model itself can integrate mechanisms to amend biases in the way it works.
An example of how this issue has been addressed by BBVA Data & Analytics with its own models is “Reinforcement Learning for Fair Dynamic Pricing”. In this article, BBVA data scientists construct a dynamic price-setting process using an AI model that incorporates “principles of justice based on fairness” to avoid discrimination. In the work, they propose a metric aimed at gauging “how fair” real-time pricing policies are and to include these measures in the optimization process. This allows you to “control and audit the fairness of the algorithm at any point”, Maestre explains.
External organizations
Apart from in-house work by scientists to ensure the quality and transparency of their models, there are also a number of organizations working to define basic principles to guide institutions to come up with more ethical algorithms.
In the United States, there are different associations that bring together the university campus with civil activism - such as AI Now in which New York University and the Algorithmic Justice League with the help of MIT Media Lab - raising their voice to warn about the power of algorithms. There are technological companies themselves such as Microsoft with its initiative Fate (which stands for Fairness, Accountability, Transparency and Ethics) studying the possible social dysfunctions of artificial intelligence.
"Not only the answers, but also the questions we ask, should be based on ethics"
According to many, the solution lies in greater transparency. They ask for a “full light of day” approach in the sense of citizens having the right to know if an algorithm can decide on something that concerns them while at the same time requiring information on the formula for the algorithm.
BBVA is involved in the TuringBox project, an initiative also backed by MIT Media Lab, that fosters the study of the “behavior” of AI systems. The project lays out a testing ground to assess the properties and effects of different AI systems to see how they behave. The web provides the option of uploading algorithms to the platform where different testers can assess how it works based on different metrics.
The role of ethics
But who decides what is biased or not? That depends on the type of bias being examined. “The motives behind unfair discrimination are generally defined at the highest level in the body of law; constitutions of countries prohibit discrimination on the basis of gender, race, beliefs, sexual orientation etc,” says Maestre. These norms help scientists establish the patterns to measure in judging if the behavior of an algorithm is “fair” or not.
However, there are other biases not included in these guidelines and, therefore, not measured. “A lot of the time, it is relatively easy to identify biases but not so when it comes to measuring them correctly,” Maestre explains. “And depending on the problem, determining whether a bias is positive or negative is no easy matter since it depends on the point of view of the person you ask”.
The key for Maestre lies in the ethics in which not only the answers but also the questions asked of the data should be steeped. “It is our duty to be aware, control and mitigate the biases existing in the training data, and refrain from using artificial intelligence to widen preexisting divides”, according to Maestre and other experts in a recent article on the responsible use of data and algorithms.