From neuroscience to banking
Obsessive Compulsive Disorder is a neurologic condition that causes people suffering from it to be dominated by anxiety and develop unwanted thoughts that they cannot get rid of. Researchers at the Universidad Carlos III are applying artificial intelligence techniques to detect which brain areas are affected by this disorder. Emilio Parrado, a data scientist at BBVA was one of the researchers that took part in the project and explains how machine learning can bring huge improvements to any activity, including finance.
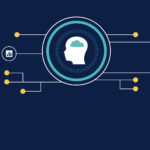
Emilio Parrado explais that OCD is a disorder that affects roughly 3% of the population, and which can have a tremendous impact on the lives of those who suffer from it, to such an extent that in some extreme cases even the simplest things like going out for a walk become a real challenge.
A Telecommunications Engineer, Emilio has spent twenty years of his life working as a machine learning researcher. Although his academic background has nothing to do with medicine, while working as a professor at Universidad Carlos III he had the opportunity to take part in a research study on OCD, together with a consortium of hospitals in Barcelona, which brought him closer to the world of neuroscience.
"The purpose of this study was never to find a new or revolutionary method to diagnose OCD. It focused on expanding the knowledge that we have today about the disease," explains Parrado. The project built on previous studies which had suggested that there existed a structural differences in certain brain areas of OCD patients. Based on this hypothesis, they decided to apply image recognition and artificial intelligence technologies to analyze the MRIs of 172 patients.
Using automatic learning techniques and an algorithm specially developed for this project, they managed to isolate the information relevant to the study and identify the different brain areas that were affected by the disease. The analyzed MRIs showed that there were indeed significant differences that appeared as "blank gaps" in OCD patients.
The key result of the study was the identification of 55 brain regions that could be related to OCD. In fact, it found that by focusing only on these regions instead of on full MRI scan, classification errors were reduced by 30%. In Parrado’s own words, "the project was not to diagnose or find a definitive solution", however the results obtained will be used in later clinical studies.
The study's main output was to increase the level of accuracy we have in identifying OCD related areas of the brain. Thus, a door was opened to future research studies where the focus is on developing specific treatments for patients with anomalies in specific regions of the brain affected by the illness.
The leap to the corporate world
Four years ago, Parrado changed his position as university researcher for finance, where he also takes on "important and exciting challenges" that put his scientific curiosity to the test. At BBVA, he is applying his artificial intelligence and machine learning expertise to improve algorithmic 'trading' strategies in securities markets.
As for seemingly non-existent similarities between the neuroscience project and his day to day role at the bank, for Parrado "in the end it is about working with data to answer questions that arise in any type of field."
Juan José Tébar - Data Scientist Team Leader for Banking at BBVA - who works closely with Parrado, argues that this is the reason why the bank needs people with scientific profiles, "because they have precisely the capacity to work with the large volumes of data that we have to the questions that our clients and the business areas ask us."
In this sense, Tebar highlights that, in addition to very specific skills in statistics, mathematics, machine learning and natural language ... etc, and a background in programming and databases, data scientists need to have a very clear understanding of the business well and know how to communicate results in an easy way so that business units can understand what the analytics is conveying.
"These are the essential elements that make a data analytics project succeed or fail," he concludes.