Deep learning and machine learning: key differences between the two big brains of the digital age
Deep learning is a subset of IA within the broader concept of machine learning, so both concepts have a good deal in common, though also differences. Deep learning uses neural networks to draw complex conclusions in a human-like manner. Meanwhile, machine learning is able to make predictions from patterns, although it requires a higher level of human input.
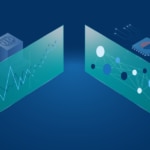
Artificial intelligence is present in various fields, such as in chatbot systems to improve customer service, in cybersecurity strategies, or in the production chain of various businesses. This broad technology features two key subsets in its development: deep learning and machine learning, which present some key differences.
“It is important to note that deep learning (DL) is a subset of machine learning (ML), so when we talk about DL, we are referring to a more specialized type of ML, whose architecture is based on more complex neural networks,” explains Montserrat Sacie, data scientist at BBVA AI Factory.
To understand the differences between artificial intelligence, machine learning and deep learning, we can use the analogy of a Russian tea doll (matryoshka), proclaims Daniel González Medina, professor on the Master’s Degree in Data Science and Big Data at IEBS business school. The larger doll would represent artificial intelligence, a broad field devoted to the creation of machines able to mimic human capabilities. Within AI, we have machine learning, a branch focused on techniques that allow machines to learn to perform specific tasks from data. The smallest doll would be deep learning, which attempts to mimic the way humans think and learn.
Delving into deep learning and machine learning
To identify the differences between deep learning and machine learning, we need to take a deeper dive into the functioning of these disciplines:
- Machine learning. Masterful in pattern recognition and in making predictions and recommendations by processing large quantities of data. “It’s like if you have a flashlight that turned on whenever you said ‘it's dark,’ so it would recognize different phrases containing the word ‘dark,’” Zendesk, a US software company, gives as an example.
- Deep learning. This particular model is designed to analyze data and draw human-like conclusions, relying on a layered algorithm structure known as an artificial neural network.
“Neural networks, which are modeled after the human brain, are made up of thousands or even millions of densely interconnected single processing nodes organized into layers of nodes,” the authors explain in a paper published by the Massachusetts Institute of Technology (MIT). Therefore, going back to the example of the Zendesk flashlight, this tech will extend its automatic capabilities: “With a deep learning model, the flashlight could learn to understand that it should turn on with the cues ‘I can't see’ or ‘the light switch is not working,’ possibly combined with a light sensor.”
Thus, the main difference between the two technologies, according to Google, is that deep learning “requires less human intervention.” “Essentially, deep learning can learn from its own errors, while machine learning needs a human to intervene,” they say. As a result, deep learning also requires much more data and computational power. “Machine learning can typically be done with servers running CPUs, while deep learning often requires more robust chips such as GPUs,” they add.
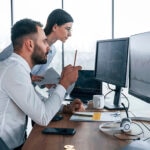
Deep learning and machine learning: in which context is each of them used?
Due to the specific traits of deep learning and machine learning, they are used for different types of tasks. Montserrat Sacie of BBVA AI Factory explains the kind of operations for which each of these disciplines is best suited:
- Machine learning. This technology is best suited to regression problems, such as predicting future account balances, and classification, although also for ‘clustering’ (grouping of unlabeled data), such as classifying customers into groups according to their financial interests, for example, “to pay off debt”, “to save” or “to invest.” Another use is time-series forecasting, such as for future trends in stock prices, and, in general, simple algorithms based on the use of mathematical and statistical rules for data processing. For example, the possibilities of machine learning are being used by researchers for tasks such as predicting earthquakes.
- Deep learning. This technology excels at handling large quantities of unstructured data and where the relationships between them become more complex. Notably, complex or deep neural networks (DNN) are applied in image processing, speech and audio recognition and natural language processing (NLP). For example, language models are used to analyze the written feedback sent by customers to find relevant topics they talk about, as well as their level of satisfaction. It can also be used to accelerate research into technological materials such as electric vehicles, solar cells and microchips, as demonstrated by Google’s AI tool DeepMind.
According to Montserrat Sacie, deep learning also happens to have experienced a remarkable boom in recent years due to the introduction of models such as GPT, developed by OpenAI, and BERT, created by Google. This has driven trends such as ‘transformer models’ (neural networks that learn by context) and large-scale language models (LLMs), such as GPT-4 and Gemini, which have generative artificial intelligence capabilities to create text or images in response to specific commands.
Deep learning represents the beginning of a hypothetical future in which, according to some experts, AI could become more adept than the human brain. This scenario is known as artificial superintelligence and, although it is currently a theoretical concept, it demonstrates that current models, however advanced they may seem, still have a long way to go to reach their full potential.