The BBVA Foundation bestows its award on the architect of the first machines capable of learning the way people do
Geoffrey Hinton’s work at University of Toronto is focused on a branch of artificial intelligence called deep learning. His goal is to develop a new breed of computers capable of learning in the same way as the human brain. At Google, where he has also been working for a number of years now, he has been instrumental in the development of a number of applications, including automatic translation system, driverless systems or tumor malignancy classification.
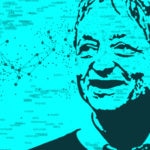
The BBVA Foundation decided to bestow the Frontiers of Knowledge Award in the Information and Communication Technologies on Hinton as a recognition of “his pioneering and highly influential work to endow machines with the ability to learn”, in the words of the jury’s citation. The new laureate, the jury continues, “is inspired by how the human brain works and how this knowledge can be applied to provide machines with human-like capabilities in performing complex tasks.”
The human brain, the best learning machine we know
The deep learning field that owes so much to Hinton’s expertise is described by the jury as “one of the most exciting developments in modern AI.”
“The best learning machine we know is the human brain. And the way the brain works is that it has billions of neurons and learns by changing the strengths of connections between them,” Hilton explains. So, one way to make a computer learn is to get the computer to pretend to be a whole bunch of neurons, and try to find a rule for changing the connection strengths between neurons, so it will learn things like the brain does.”
Hinton is Professor of Computer Science at the University of Toronto, and, since 2013, a Distinguished Researcher at Google, where he was hired after the speech and voice recognition programs developed by him and his team proved far superior to those then in use.
His research has since speeded the progress of AI applications, many of them now making their appearance on the market: from machine translation and photo classification programs to speech recognition systems and personal assistants like Siri, by way of such headline developments as self-driving cars.
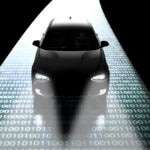
Self-driving car.
Self-driving family cars in five-years’ time
Biomedical research is another area to benefit – for instance, through the analysis of medical images to diagnose whether a tumor will metastasize, or the search for molecules of utility in new drug discovery – along with any research field that demands the identifying and extracting of key information from massive data sets.
Asked about the deep learning applications that have most impressed him, he talks about the latest machine translation tools, which are “much better” than those based on programs with predefined rules.
He is also upbeat about the eventual triumph of personal assistants and driverless vehicles: “I think it's very clear now that we will have self-driving cars. In five to ten years, when you go to buy a family car, it will be an autonomous model. That is my bet.” In Hinton’s view “machines can make our lives easier, with all of us having an intelligent personal assistant to help in our daily tasks. They will be extremely useful.”
Developing machines that can drawfrom their own experience to learn
Deep learning draws on the way the human brain is thought to function, with attention to two key characteristics: its ability to process information in distributed fashion, with multiple brain cells interconnected, and its ability to learn from examples. The computational equivalent involves the construction of neural networks – a series of interconnected programs simulating the action of neurons – and, as Hinton describes it, “teaching them to learn.”
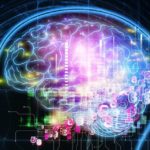
The eminent scientist’s research has focused precisely on discovering what the rules are for changing these connection strengths. For this, he affirms, is the path that will lead to “a new kind of artificial intelligence,” where, unlike with other strategies attempted, “you don’t program in knowledge, you get the computer to learn it itself from its own experience.”
In the case of artificial neural networks, what strengthens or weakens the connections is whether the information carried is correct or incorrect, as verified against the thousands of examples the machine is provided with. By contrast, conventional approaches were based on logic, with scientists creating symbolic representations that the program would process according to pre-established rules of logic.
“I have always been convinced that the only way to get artificial intelligence to work is to do the computation in a way similar to the human brain,” says Hinton. “That is the goal I have been pursuing. We are making progress, though we still have lots to learn about how the brain actually works.”