Algorithms that see better than humans: this is how facial recognition works at BBVA
The branch of artificial intelligence that focuses on teaching machines how to see is becoming increasingly sophisticated. It is already better than humans at telling one person from another. At BBVA’s headquarters, this technology is already being used to allow users to pay for their meals at Ciudad BBVA’s restaurants. But, how does it work?
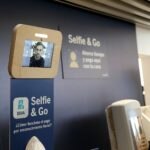
Artificial vision (or 'computer vision') is the technology that allows a computer to interpret the information contained in an image, for example, to recognize and extract data from a credit card or an ID card when scanning it. This is the type of technology that allows Facebook to tag you in photos or Snapchat to tell where an individual’s face is when applying the puppy filter. Artificial vision is also the technology that allows self-driving cars to see what’s on the road and navigate around obstacles.
In short, computer vision is a technology allows creating applications capable of using data from camera pixels to identify objects and interpret spaces. The most basic of the technology’s virtually endless range of applications have been in use for decades. But in recent years, major progress in machine learning has helped spawn a new breed of solutions capable of handling increasingly complex tasks, many times more accurately than a human would. One example of this is the biometric recognition system rolled out in June 2018 at Central restaurant – located in Ciudad BBVA, the bank’s headquarters in Madrid - to streamline the payment process. This system allows users to pay just by setting their trays on one of the stands located next to the cash registers and looking at a screen. At each stand, an AI-powered system identifies the customer’s face – provided that they have registered in the system -, and the food on his/her tray and automatically processes the corresponding payment, debiting the customer’s registered credit card.
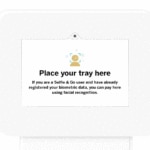
The system recognizes the user’s face through cameras equipped with artificial intelligence, and directly charges the card associated with their profile.
But, how can the system tell if the person is who they say they are? "When we talk about artificial intelligence, we are talking about replicating human intelligence through automated means, using machines capable of making decisions or inferring results from the reality they capture, just as we do," explains Mikel Sánchez, CTO of Veridas, the company responsible for the development of the technology enabling the aforementioned application. BBVA, in partnership with Pamplona-based company Das Nano, founded Veridas in 2017 to speed up the development of more secure and user-friendly customer identification and authentication systems.
In this case, what the machine does is compare the facial features of the person initiating the payment process against those of all the people "allowed pay", i.e. the system’s registered users. After finding a "match", and identifying the menu on the tray, it will process the corresponding payment by debiting the user’s registered credit card. The entire process is completed in a matter of seconds.
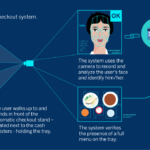
From algorithms to neural networks
The use of artificial neural networks has boosted the capabilities and accuracy of this type of systems in recent years. Before, they used algorithms based on complex mathematical formulas aimed at determining the "theory" what a face looks like, the parameters the system had to identify to determine if what analyzing was a face. Today, they learn through practice. "With the development of neural networks, the key to problem-solving lays not so much in algorithms but in the data," says Carlos Arana, CTO of Das-Nano, the company that developed the food tray-recognition technology enabling the BBVA system.
"It is the network itself that deducts what it should pay attention to in order to distinguish one face from another”
What neural networks do is mimic human learning processes, using a system that automatically processes thousands, or millions of parameters from different faces and trains itself in learning how to tell them apart. “We tell the system: these two faces belong to the same individual, over and over again until it learns how to distinguish them from the rest of the database," adds Sánchez. Rather than trying to match for a specific set of individual features, the system learns how to recognize each face’s set of features as unique. "What makes a person’s face recognizable is not a nose, or a pair eyes, but the combination of features as a whole," he adds. Similarly, humans recognize faces based on the sum of features, not just on a set of them.
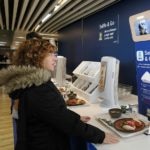
The system has already processed over 10.000 meals.
Ultimately, a system "reads" information, i.e. the ones and zeros in each pixel that makes up an image. The system does not "care" whether this information comes from a mouth or an ear. "When training a neural network to recognize faces, it is not told to focus on a number of points or specific features. Much like children are not taught to tell people apart based on points of reference. It is the network itself that deducts what it should pay attention to in order to distinguish one face from another," says Sanchez. What the system therefore looks for are coincidences between different data sets in each face, based on which it can determine whether they belong to the same arrangement of pixels and, therefore, to the same features in a face.
Thanks to the computational capacity of current systems and the increasing availability of data for training purposes, these systems have become exponentially more accurate in recent years and started outperforming other market technologies in a short period of time. In fact, facial recognition systems capable of analyzing the similarity between two faces to determine whether they do or do not belong to the same person, and are already beating humans at it.
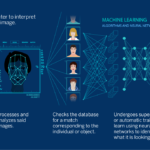
Whether for recognizing faces, voices, food, documents or any other image, the power of neural networks lays in having appropriate data sets that can be classified and used to train the models correctly "so that they are capable to infer the answer, just as any human would," Sánchez explains.
A pioneering system
BBVA’s quick checkout system is equipped with a camera for recognizing users and another one for recognizing that the customer has a menu on his/her tray, in order to process they payment. While feature patterns in faces are similar, the items on the tray vary enormously: BBVA’s restaurant offers more than 15 different menus, and each person is free to combine elements to their liking.
From a technological standpoint, the system’s development was "a real challenge," which the experts were able to solve thanks to a combination of skills, ingenuity and huge amounts of datasets. On top of the images captured at the restaurant, they trained the system using over two million "synthetic" pictures they took, consisting of trays with different products arranged differently on them. "We arranged the items randomly in a small studio combining all sorts of color and element variations to create more examples to train and strengthen the system."
"The work allowed us to push a bit the limit of what is being done in terms of recognizing this type of images," says Arana, who describes the system’s development as “a great learning experience” for the different teams involved, as it focused on a very complex use case, in which they "pushed the system to its limit" keeping user experience intact.
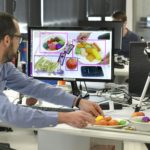
They trained the system using over two million pictures of trays with different products arranged differently on them.
The system has already processed over 10,000 payments, allowing hundreds of employees to enjoy their meals more comfortably, speeding up checkout times by up to five minutes, and saving them the trouble of going through a manual payment system that required them pulling their cards or cash out.
Finally, with these new solutions retailers can start offering direct rewards to their customers – subject to their previous consent - through the 'app', such as complimentary coffee with their meal, or discounts after a specific number of orders (pay for nine, get a tenth one for free!).
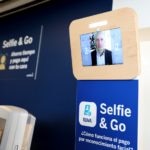
The system speeds up checkout times by up to five minutes.