Algorithmic fairness as a key to creating responsible artificial intelligence
Artificial intelligence is increasingly mainstreamed in society and used to make decisions that affect people´s lives in areas such as their workplace or the justice system. This presents the challenge of biased algorithms, which are mainly caused by biases or distortions in the training data. Proposals are already in place that caution against bias and seek to mitigate its harmful impact on society.
Opening imagen created with Midjourney (AI)
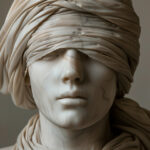
More than 30% of large companies in the European Union now use artificial intelligence (AI). This trend reflects how AI has permeated various aspects of life, from employee recruitment to product sales and cybersecurity. Behind these algorithms, however, lies one of the biggest challenges of AI: biases. Finding mechanisms to mitigate bias and achieve algorithmic fairness has become a necessity to build models aligned with agreed human criteria.
"Algorithmic fairness consists of designing and developing artificial intelligence (AI) systems, including machine learning (ML) systems, that operate in a fair and equitable manner and do not discriminate," explains Marco Creatura, data scientist at BBVA AI Factory. "The main concern is that AI should not replicate, reinforce, or amplify existing social biases. Fairness, in this context, implies the absence of bias. In the field of decision-making, bias is defined as any prejudice or favoritism toward an individual or group based on their inherent or acquired characteristics," he continues.
Moreover, generative AI algorithms are more complex than classical 'machine learning' algorithms, whose output is usually a score or a probability. “Large language models are trained on vast amounts of textual data, typically sourced from the Internet. This data is often uncurated, meaning it can include stereotypes, misrepresentations, and exclusionary or derogatory language about certain social and vulnerable groups. The complexity lies in the fact that language is itself a technology that mirrors social and cultural norms," says Clara Higuera, data scientist at BBVA AI Factory. Bias detection in generative AI is a nascent field that is still in its exploratory stages. Despite this, it is already being applied to create guardrails and tools that identify these biases.
In fact, according to a UNESCO study, the language models used by generative AI can reproduce gender, racial and homophobic biases that contribute to misinformation.
How do biases, the major obstacles to algorithmic fairness, come about?
Biases are manifold and can show up at different stages, as noted in an article in MIT Technology Review:
- During the problem definition stage. Developers start by establishing the goal of the algorithm they are building. This involves defining metrics out of diffuse aspects such as 'effectiveness,' a fuzzy and subjective concept open to interpretations that are not always impartial. For example, if a streaming content platform's algorithm seeks to maximize viewers' viewing time, this could result in recommendations that reinforce their previous interests rather than diversify their experiences with other content.
- During data collection. There are two possible reasons for this phenomenon: either the data collected are not representative of reality, or they reflect existing biases. For instance, if an algorithm receives more photos of light-skinned faces than dark-skinned faces, facial recognition will be less accurate in the latter case. Another example is what has happened with some hiring tools, which, for instance, ruled out women for technical positions because the algorithm was trained on historically biased hiring decisions.
- During data preparation. Attributes that the algorithm will use to make decisions, such as age or personal history, are often selected and prepared beforehand. Such attributes may bring socio-economic or gender-related biases into AI systems. These biases can also creep in when labeling data that will later be used by algorithms, especially when performing data annotation tasks. This is because annotators may bring their own biases, hence the importance of writing clear annotation guidelines.
What kinds of bias should algorithmic fairness address?
The biases that algorithms can exhibit come in a variety of forms and types. "Addressing and mitigating bias is essential to developing AI systems that are fair and beneficial to all users. We thereby ensure equitable decisions and build trust in emerging technologies," say Marco Creatura and Clara Higuera. Some types of bias, according to Google, include:
- Selection bias. Selection bias arises when examples from a data set are chosen in a way that does not reflect their distribution in the real world. "Given that you can't train an algorithm on the entire universe of data, the subset must be carefully chosen based on this context. A sample that is not representative of the whole, or one that is unbalanced towards one group, will lead to equally biased results," the Cervantes Institute explains.
- Automation bias. This is the tendency to believe by default everything that automated systems report regardless of actual error rate. This leads to information being taken for granted that sometimes may not be fully substantiated. "When people have to make decisions in relatively short time frames, using sparse information...that's when they tend to rely on whatever advice an algorithm gives them," notes Ryan Kennedy, a University of Houston professor specializing in automation, in a research paper.
- Correspondence bias. Correspondence bias arises when algorithms generalize about people, evaluating them based on their group membership instead of assessing their individual characteristics. For example, by assuming that all people who attended the same college are equally qualified for a job.
- Implicit bias. Implicit bias arises when assumptions are made based on the personal situations and experiences of the algorithm developers themselves, which do not apply at a more general level. This bias could influence their approach to modeling and training, as developers may introduce their own biases inadvertently.
Initiatives and proposals to achieve algorithmic fairness
There are many more types of biases that may be present in the algorithms that society interacts with on a daily basis. But there are also initiatives and regulations aimed at promoting algorithmic fairness and mitigating situations of injustice. "Governments and organizations have started to implement guidelines and regulations to ensure that AI technologies are fair and responsible. This includes the creation of ethical frameworks and specific legislation on the use of AI to protect against discrimination," says Marco Creatura, who also points to the European Union's Artificial Intelligence Regulation (AI Act).
In fact, the European Union also runs a project that provides proposals and templates for companies to audit whether systems and applications comply with the General Data Protection Regulation (GDPR) and with various transparency requirements. In this way, organizations ensure that they rely on best practices for trust and security, as one of Europe's goals is to ensure that AI works for all people in an inclusive manner.
In Spain, the Spanish Data Protection Agency (AEPD) has published a set of guidelines for auditing AI systems with a specific section for checking for bias in the data sources used. "Current research focuses on methods to correct for biases in data and models. This includes more representative data collection techniques, adjustments to algorithms and modifications to the post-processing of results to ensure fairer decisions," says Marco Creatura.
Furthermore, fairness metrics have emerged to evaluate machine learning models. There is an emphasis on making AI models more transparent and multidisciplinary. Such models involve not only data scientists and developers, but also ethicists, sociologists and representatives of affected groups. This can be seen through projects such as the Algorithmic Justice League, an organization founded by Joy Buolamwini, a researcher at the Massachusetts Institute of Technology (MIT) and pioneering AI activist. This institution is dedicated to analyzing how AI systems can lead to discrimination in different ways, and informing the public about the risks. As they state on their website, although the new tools are promising, it is crucial to build "a movement to shift the AI ecosystem towards equitable and accountable AI.”
There are also techniques designed for generative AI algorithms such as guardrails. “These are guidelines and tools for monitoring, evaluating and guiding the behavior of generative AI models. They can range from an instruction in the prompt (e.g. “answer politely and respectfully without insulting anyone”) to models that detect when a response may contain hate speech to be avoided," says Clara Higuera.
In addition, business has an important role to play in the development of unbiased AI. In fact, in the case of BBVA, algorithmic fairness is a priority as a principle of responsible AI. The Bank is therefore working on the development and implementation of practices that promote fairness and non-discrimination in artificial intelligence systems across the Group.
Achieving algorithmic fairness is a crucial challenge that has yet to be addressed. "This is a major issue that we are working on. We need to raise awareness at all levels involved in the AI development cycle, from senior leadership to data scientists. This way, we can develop genuinely fair systems," Clara Higuera stresses. Advances in research, regulation, and collaboration to ensure that algorithms treat everyone fairly will help reduce bias and promote equity.