Frontiers of Knowledge Award for laying the foundations of modern Artificial Intelligence
The BBVA Foundation Frontiers of Knowledge Award in Information and Communication Technologies has gone in this fourteenth edition to Judea Pearl for “bringing a modern foundation to artificial intelligence”. The Professor of Computer Science at the University of California (UCLA), has made contributions that enable AI programs to use two of the key resources we humans use to interpret the world and arrive at decisions: probability and causality.
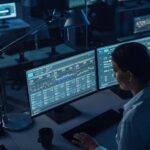
“By laying the mathematical foundations for probabilistic reasoning and the inference of causal relationships, Pearl constructed a framework not only for computer-based thought, but for fields spanning computer science, mathematics and statistics, epidemiology and health, and the social sciences", stated the citation.
Pearl explains his contribution to understanding probabilistic reasoning in the human brain so that it can be replicated in a computer system: “Uncertainty is the prevailing raw material in everyday decision making, even when crossing the street, taking an aspirin or talking to friends; we have a hard enough time getting a computer to deal with the barrage of noise and uncertain information it has about the world. My work has developed a calculus for probabilistic reasoning that allows the computer to handle all the kinds of noise information that it gets, put them together, and come out with a probability about the conclusions.”
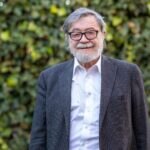
Judea Pearl, BBVA Foundation Frontiers of Knowledge Award in Information and Communication Technologies - BBVA Foundation
In an interview shortly after hearing of the award, Pearl summed up what he considered to be his essential contribution to modern AI: “For the first time we can understand what ‘understanding’ means, for the first time we have a computational model of deep understanding.” Pearl defines such understanding as “being able to answer questions of three important levels: prediction [what will happen in such and such a circumstance]; the effect of actions; and explanation, why things happened the way they did and what would have happened had things not occurred as they did. These three levels of sophistication are what the language now captures and that is what we mean by an understanding.”
Pearl’s candidature was supported by 11 eminent researchers in Europe and the United States. Among them is the Nobel laureate in Economics Daniel Kahneman, who says of his nominee: “During my long career I have known quite a few scholars who were recognized as giants in their field. I do not think I ever encountered one who evoked as much reverence and as much affection as Judea Pearl.” Another of the nominators, Vinton G. Cerf, Vice President and Chief Internet Evangelist at Google (United States), recalls how he has “watched in admiration as his work has blazed new trails, often against conventional wisdom. Judea Pearl pressed on in his research in the face of considerable skepticism and his efforts were soundly rewarded.”
A “less opaque” AI
Nominators are keen to emphasize that, compared to other lines of AI and statistics like deep learning or neural networks, Pearl’s contribution lends a transparency that is vital in determined applications, such as decision-making in medicine and on legal or economic issues.
Pedro Larrañaga, Professor of Artificial Intelligence at the Universidad Politécnica de Madrid (UPM) has, over three decades, made a theoretical study of Pearl’s contributions, in areas such as bioinformatics, neuroscience and industry. As he sees it, the awardee’s insights facilitate a “less opaque” AI that reveals by what reasoning intelligent systems have arrived at a given conclusion: “Many of the recent breakthroughs in AI have been based on neural networks that employ deep-learning algorithms. But these programs are like black boxes, where it’s not possible to trace how they came to solve the problem. Lined up on the other side are those of us who work habitually with Pearl’s Bayesian networks, in the belief that interpretability has to be a cornerstone of AI.”
Getting machines to successfully detect causal relationships can unlock multiple applications. “Now we have a language to do that, so we can insert the knowledge that we have about the world and infer coherently like we do in algebra,” Pearls elaborates. “We infer the conclusion and the conclusion is proven to be correct if the assumptions are correct. The applications go from personalized medicine to handling an epidemic like COVID, putting together a variety of information sources from various parts of various countries, and coming out with a coherent conclusion based on the evidence that we have.”
On this point, Larrañaga relates how his team has supported health centers during the pandemic by applying Bayesian networks to decisions on which patient should be intubated, and in predicting the course of the disease: whether ICU admission would be required, or the length of hospitalization.